Short summary from the ESTAD 2017 talk session, June 26th-29th, Vienna
M. J. Neuer
Digitization is a key enabling technology for the European process industry. It helps to maintain a leading position with respect to product quality, but also to push the efficiency limits, reliability and quality to a next level. Recently, many steel companies updated and overhauled existing infrastructures and prepared for being “Big Data-ready” and for applying “Industry 4.0” [1,2] concepts.
Yet, the practical work to gain benefits from the new technologies is far from being easy [3,4]: Some riddles of the process plants are: How can blurry Industry 4.0 concepts be applied to current, very real problems? What advantages do they have and what must be done to achieve economic impact from using the new digital resources?
Data Retrieval in the Brown-Field Scenario
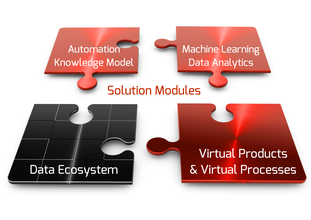
Data is an important resource. This resource is currently not used efficiently due to several hindrances. On one hand, data infrastructures of production facilities
are often very heterogeneous, as they naturally grow with a company’s IT demands. We are confronted with a so-called brown-field scenario. Industrial plants therefore must support old and
outdated hard- and software systems, as well as carefully expand towards novel technologies. Having this in mind, introducing Industry 4.0 means to successfully integrate and support the existing
industrial IT infrastructure. Agents connect to databases, sensors and actors, by using a semantic model of the plant
[5]. With this approach, they can be safely integrated into arbitrary IT landscapes, where they act autonomously and without interfering with the plant processes. The semantics introduces
a self-awareness of the agent for its environment.
NoSQL
Another important pillar of our approaches are Not-only-SQL (NoSQL) databases, which are a preferred solution to manage product-oriented data. Each product is here considered as an object, being filled up with process data as the real product travels through the processing steps. In practise, we use a Python framework for linking to nearly any existing database technology. This includes common SQL systems as used in process industry, but also the above-mentioned NoSQL databases, considering Apache CouchDB, MongoDB, MariaDB or Google BigQuery just as some renown examples. Agents were developed to ease the work of talking to the databases and they provide simple and reusable services within the network. Such a data management can be retrofitted to any existing IT and database technology.

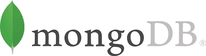
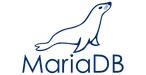
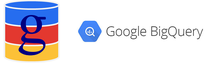
Machine Learning
The Betriebsforschungsinstitut BFI develops machine learning and data analytics solutions for steel industry, always adopting a process oriented perspective. Several years ago, when data mining started to provide new insights into process understanding, it was rolled out for use in quality departments. But those former investigations and quality analyses restricted to specific sub-processes. Moreover, highly aggregated data such as average values or minima and maxima were used for establishing predictors, due to limited data availability or processing constraints. For important problem classes, decisive information was lost in the aggregation.
Today, with modern technologies in place, this situation has tremendously improved. A new generation of data analytics is today directly applied to fully resolved process data. Quality prediction can be done online, close-to-process and in near-real-time. In-house developed tools for data analytics are a first building block of this solution module. Transforming abstract and large data volumes into human understandable form.
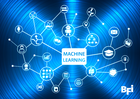

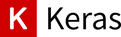
Automatic Causality Detection
We also see that reliable, automatic detection algorithms for specific process situations and their causal interrelationships play a key role. They extract sets of process relevant shapes from signals and uses them for feeding data analytics algorithms as e.g. random forests. We regard to this approach as process-based feature extraction. This is also strictly different from other approaches originating from pure IT perspectives, where data piped through analysis algorithms without respecting the physical nature of the process.
Bibliography
[1] Bundesministerium für Bildung und Forschung: Zukunftsbild „Industrie 4.0“. Berlin 2013
[2] Michael Chui, Markus Löffler, Roger Roberts: The Internet of Things. in: The McKinsey Quarterly
[3] M. J. Neuer, A. Ebel, A. Wolff, F. Marchiori, M. Roeßiger, N. Matskanis, G. Mathis, Dynamisches Umplanen von Produkten führt zur Verringerung von Ausschuss und verbessert den Gewinn, stahl und eisen 135 (2015), Nr. 11
[4] M. J. Neuer, A. Ebel, A. Wolff, F. Marchiori, M. Roeßiger, N. Matskanis, G. Mathis, Raising economic efficiency of steel products by a smart re-allocation respecting different process routes, Proc. European Steel and Application Days (ESTAD) 2015, Düsseldorf
[5] S. Zillner, A. Ebel, G. Mathis, M. Schneider, L. Abele, N. Goldenberg, “A Semantic Modelling Approach for the Steel Production Domain”, 1th European Steel Technology & Application Days & 31th Journees Siderurgigues Internationales (JSI), Paris, 04/2014
[6] A. Ebel, N. Link, “Knowledge based decision support system for slabs approval at the steel shop”, 1th European Steel Technology & Application Days & 31th Journees Siderurgigues Internationales (JSI), Paris, 04/2014
[7] M. J. Neuer, A. Ebel, F. Marchior, N. Holzknecht, J. Brandenburger, How to optimize steel quality by applying Industry 4.0 techniques in real-world practical examples, European Steel Technology and Application Days (ESTAD), 2017, Vienna
[8] M. J. Neuer, M. Loos, D. Sonnenschein, J. Polzer, Economic impact maximization and throughput increase by process self-optimization applied to a pickling line, European Steel Technology and Application Days (ESTAD), 2017, Vienna